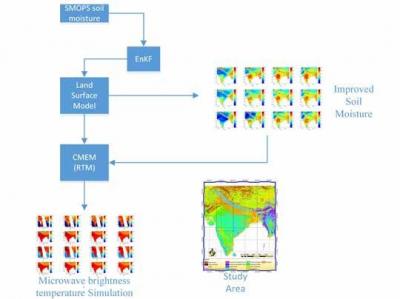
India supports around 17.5% of the world’s population on a mere 2.4% of the Earth’s surface prone to global and regional climate change effects. Scientific research has largely focused on using models as inseparable components of climate studies. For a genuine understanding and realistic representation by which land surface processes influences climate, the role of land surface models (LSM) can never be overstated. Land surface states (like precipitation, soil moisture and temperature, soil moisture) represent one of the crucial factors which govern the global and regional climate through the exchange of moisture and energy between different land cover classes and the overlaying atmosphere.Amongst all the land surface states, soil moisture estimates play a crucial role in moderating climate on a seasonal and annual timescales. Even though soil moisture represents a teeny tiny slowly varying component of the earth system, it sits at a highly critical zone on the land surface thereby playing a crucial role in the global water cycle. For this same reason, prediction of soil moisture dynamics poses a high priority research challenge. The sensitivity of microwave remote sensing particularly at L band (1–2 GHz) and C band (4–8 GHz) have triggered satellite missions for soil moisture measurement from space like AMSR-E (advanced microwave scanning radiometer - Earth observing system), SMOS (soil moisture and ocean salinity) and SMAP (soil moisture active passive). The existing challenge is to make the most out of these big data which get streamedm from satellites at even sub-daily temporal scales. Our group is focused on data assimilation techniques first established by meteorologists which offers an excellent and novel means to merge data into land surface models. Our group uses this technique to exploit the increased availability of remotely sensed land surface variables for improving LSM model predictions by running in an offline and coupled mode in the fields of hydrology and meteorology. We have proved that data assimilation approaches (using kalman filter and variational data assimilation techniques) results in improved land surface simulations of soil moisture.Our research is based on the fact that these soil moisture products are rough representations of the top 5 cm of the soil column. This implies that a clearer picture of the impact of assimilation in deep soil layers is dependent on the model physics. As a direct consequence, we have developed a novel parameterisation technique over the heterogeneous southern extent of India for improved model performance.Another facet of study involves the assimilation of microwave based radar reflectivity, from space borne satellites and Doppler weather radars (DWR). We have examined the Doppler weather radar data at high temporal and spatial resolutions for improving its skill in quantitative precipitation forecasting. This is based on the hypothesis that assimilating high resolution data improves the quality of initial conditions for weather forecast models by generating an accurate image of the true state of the atmosphere at a given time. Further, our results over Western Ghats and Central Indian region indicate that the vertical structure of satellite based radar reflectivity has immense potential to study about intense convective storms. In addition, our analysis over Northern India also examines the relationship between radar and cloud-active aerosols. This scientific understanding about data assimilation of various microwave based data products (from ground based or satellite based platforms) is well needed and crucial towards their future use in accurate predictions for hydrology and meteorology at better time scales.
Prof. J Indu