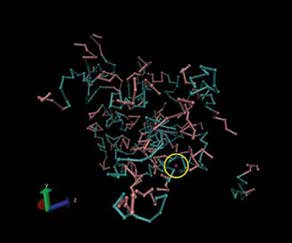
We have been involved in understanding the mechanism of transport in crowded and active medium. For this, we build statistical mechanical models and solve it either analytically or on a computer, when complicacy of the problem does not allow exact analytical solutions. Availability of single molecule data also makes it possible to compare our results with experiments. A realistic example would be biological cell where presence of cell organelles makes it highly crowded. In addition, several adenosine triphosphate (ATP) induced active processes take place inside the cell and hence dynamics of biomolecules inside a biological cell can be classified as ‘dynamics in crowded and active medium’.
Passive and active tracer dynamics in polymeric environment
In polymeric solution, which can be considered as moderately crowded environment, dynamics of probe molecules have been found to show interesting features such as subdiffusivity and non-Gaussianity (Fig 1). Also it is not rare to encounter situations where the dynamics in non-Gaussian yet diffusive (the mean square displacement grows linearly with time). Our simple computer model can address all these and explain why is that so. More recently we are extending this to a case where the tracer is self-propelled (active) and tumbles while translating. This connects to recent experiments performed on self-propelled Janus particle in crowded medium. We hope our theoretical formulation will help in constructing self-propelled nanomotors for future drug delivery.
Colloid and single chain dynamics in bacteria bath
Bacteria run and tumble in water. This motion can actually impart additional random kicks on a colloidal particle immersed in a bath of bacteria. This makes the dynamics faster. We have built an exactly solvable model that can explain such enhanced diffusion (Fig 2). Moreover, we have extended our model in case of a polymer chain in a bath of bacteria. As expected, our model predicts faster diffusion of the chain. However, because of the presence of long temporal correlation of the random kicks from bacteria, the chain gets swelled and sustains spatial correlation for longer time. This results in slower reconfiguration of the chain in bacteria bath. We are now currently engaged in building a theoretical framework for a micro-sized machine operating between bacteria baths.
Prof. Rajarshi Chakrabarti