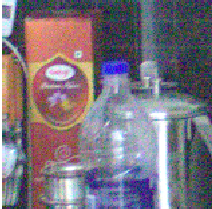
In today’s world, images are ubiquitous, from the photographs we take, the videos we watch on youtube, and the images such as XRays, CT (Computed Tomography) or MRI (Magnetic Resonance Imaging) scans acquired in a hospital to satellite images. These images form an important tool for visualisation or representation of data. Our group has been working on a variety of algorithms for processing such image data to improve their appearance and to make them more useful, which also includes acquiring them faster A few highlights of the work we have been engaged in are
presented here below:
Owing to various factors such as poor lighting or issues with sensing elements, the images acquired by a camera may appear grainy. These grainy artifacts are often called ‘noise’. Noise actually is a very general term, and actually refers to unwanted components in an image. In different applications, this noise may manifest itself in different ways. If there is relative motion between the scene and the camera, the acquired image may exhibit motion blur artifacts. If the picture taken is that of a scene which is located behind a glass window, the picture contains visual disturbances due to reflections of objects located in front of the window – since the glass window acts as a semi reflecting surface. Removal of such ‘noise’ is important not only for aesthetic reasons, but also to facilitate better processing of these images for various higher level applications including recognition, surveillance and security. This process is termed noise removal or denoising.
In certain applications such as MRI or CT, the acquisition of the images can be quite time-consuming and therefore also costly as well uncomfortable or even hazardous to a patient. In fact, the images are never measured directly– they are acquired in a form loosely termed as ‘projections’ from which the actual image has to be reconstructed by a fairly complicated algorithm. The acquisition can be made faster by reducing the resolution of the projections to bring down the effective number of measurements. However this reduces the image reconstruction quality. We have been working on a number of algorithms to retain the reconstruction quality in the face of vagaries such as measurement noise in the projections, a reduced number of measurements and sensor calibration issues (i.e. the instrument acquiring the image doesn’t do exactly what you told it to do). Our algorithms exploit numerous interesting and commonly observed properties of natural images, such as sparsity and compressibility (i.e. though they contain thousands of pixels, they can accurately be represented using just a small number of ‘signature values’), or similarity among nearby regions or slices of a biological tissue. Our algorithms fall in a relatively new sub-area of the image or signal processing field called as ‘compressed sensing’.
Prof. Ajit V Rajwade