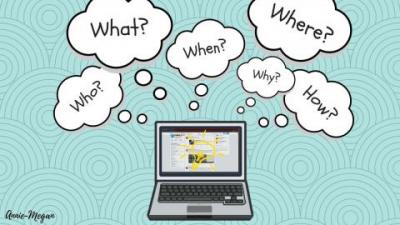
Researchers develop a search system to extract meaningful data from live social media posts
Ever tried searching ‘goal’ or ‘kick’ on Twitter during the interval of a football world cup match? You were probably trying to find out who scored the goal on a penalty kick in the first half, and chances are that search results also have content about life goals and a kick from coffee!
Numerous people post updates and messages on social media, making this text a treasure of information. Do we not turn to Facebook, Twitter or Instagram to check updates regarding recent happenings, instead of waiting for the news telecast or the morning newspaper? But being able to find the relevant information which has answers to one’s questions can be a struggle.
A team of researchers from the Department of Computer Science and Engineering, Indian Institute of Technology Bombay, in association with researchers from Microsoft India and Google, has devised a search system called Contextual Event Search, useful in extracting meaningful summaries for live events, from data streams such as Twitter, while they are happening.
Why sources such as Twitter? “We once came across a paper which claimed that Twitter can be used to identify events as and when they are happening, and gave an example of how Twitter was the first to break the news of an earthquake,” says Manoj Agrawal, member of the team which made Contextual Event Search. One significant insight which the paper brought out was that unlike other social networking platforms, Twitter is an important medium to track the real-world event as and when it is happening. This got the researchersthinking about making a general-purpose system to identify all important events using Twitter messages.
An event is an activity relevant to a group of people. For example, the final of the Football world cup or a cycle-rally to support “Go green”. A data stream is a series of social media messages, posted within a specific time period, related to an event. Since thousands of people from all over the world, post in a very short time, the information comes at a very high rate during an event and is unstructured.
It becomes difficult to discover relevant messages by the conventional keyword search, which simply returns the most recent messages, but not necessarily relevant ones. As an example, if we get to know of some mishap related to a foot over bridge at a railway station in Mumbai, we may search for ‘bridge’, ‘Andheri’ and ‘Mumbai’. Conventional search results give the address of a shop near a bridge and the location of a weighbridge and the messages informing about the mishap are buried somewhere. To understand what happened, we may have to look carefully through the search results, one by one, for the most relevant messages.
Here is how the C ontextual Event Search developed by the researchers can help. It continuously scans the Twitter data stream and automatically generates a contextual event summary for live events. It discovers important sub-events related to that particular event and arranges them in a chronological sequence. It appends additional information to this summary when there are any significant updates or progress related to that event. This database makes the contextual search easier and more accurate.
Most real-time events have several facets, or facts other than the main sequence of events, associated with it. The Contextual Event Search identifies such facets and presents a bundle of messages related to each facet, organised under the event.
Constantly updating the summary database for all the discovered events is very costly in terms of the computation resources, hence the researchers use what is called as Lazy Update Method, where summaries for only popular events are updated as they change fast. These changes are arranged so that an event thread is generated. The event thread helps in identifying and associating the messages relevant to the corresponding ‘event topic’, which is otherwise very challenging.
An important feature of this search mechanism is that it is able to discard the redundant and non-relevant data abundantly present in the social media streams. “We provide a framework to identify the important chunks from this fast-moving data stream as and when it is generated. It keeps the data size in control, making the system scalable,” explains Dr Agrawal.
Let us see how the search helps us to find details about the event “Nairobi Terrorist Attack”. The researchers demonstrated that the results returned, a set of 13 sub-events in a thread. The summary started with a tweet about a mall being attacked, followed by tweets about action against attackers, rumours, claims and counterclaims by authorities and citizens, etc., clearly the most relevant messages, which were all discovered in real time, from over 164k tweets received in that time window. If we query "Nairobi Westgate" the results include the tweet "Nearly a full day after Kenyan mall Attack began, gunman and hostage still inside the mall", demonstrating the ability to find tweets which do not have the query keywords but are still relevant to the topic. Thus, this system can be used to create a storyline for events in a live data stream.
The technology is currently at a lab scale and the researchers have demonstrated it in major scientific forums such as the International Conference on Extending Database Technology, International Conference on Very Large Databases and IIT Bombay. “Our plan is to make it more widespread. With appropriate funding to cover the cost of the resources, including the data acquisition cost for the commercial usage, and availability of human resources, we can make it commercially available in a span of 6 months,” says Dr Agrawal.